Podcast host Greg Vitarelli | Podcast guests Kevin Schawinski Co-founder and CEO at Modulos AG, Dr. Andreas S. Rath CEO & Co-Founder at Ondewo, Kuba Krchak Co-CEO & Co-Founder at The MAMA AI
June 7, 2022 | 20 min 40 sec
From Vision to Customer Integration – Conversations with AI’s Emerging Companies
In this episode, we speak with founders of fast growing AI companies Modulos AI, ONDEWO and the Mama AI whose success is based on the ability to effectively integrate their solutions with large enterprise customers.
Podcast Transcript
Welcome back to At the Qore of AI.
In this episode, we will speak with several emerging AI companies. These vendors are developing solutions to bring to their customer base. We’ll ask them about their backgrounds, company mission and the integration challenges they sometimes face when trying to scale their solutions to enterprise customers.
We’d like to welcome Kevin Schawinski, the co-founder and CEO of Modulos AG to the podcast. Kevin, welcome.
Well, thanks for having me.
Kevin, I have really enjoyed conversations with other entrepreneurs in the field of AI. What was your career path from perhaps academia through to where you are today?
Sure, yeah. So, I am by training an astrophysicist and I used to study galaxies and supermassive black holes and how they evolve and grow together. And I used to do that with data from telescopes on the ground, in space and actually, I used the machine learning or try to use machine learning techniques to analyze all that data, to get interesting scientific insights.
What was the purpose for founding the company? What problem are you trying to solve with Modulos AG?
Together with my team of researchers, we were trying to use machine learning for science. We had some really amazing results, really fascinating discoveries enabled by machine learning, but also we had a ton of trouble to get projects up and running and we really didn’t know how to make good decisions. Like what, what data do we need? What model do we need? How to make these decisions in a principled quantitative way.
So, the problem we’re trying to solve with Modulos AG is to give you that system guidance. And we’re focusing in particular on what data you really should be using to train your model because whatever machine learning model you train, it learns from training data and that training data can have errors in it, it can be broken, have missing values, it can be biased.
And machine learning is great because it learns from whatever the data is; it doesn’t have any common sense. And so if you just try and build a more and more complex model on top of bad data, you’re just going to get a bad model. And so data centric AI means actually shouldn’t spend more time on your data and Modulos AG gives you the tools to figure out where to look and what to do.
And who is the you here? What types of markets are you serving?
So we have users across a wide range of industries from metallurgy, financial services… research institutions, and we empower sort of that area in between data scientists, machine learning engineers, and some subject matter experts who understand the data but maybe don’t necessarily have such a strong background in machine learning. And so we help them collaborate on building better models, machine learning engineer to work on the model side, and then the subject matter expert to figure out what’s wrong with the data and what should be done about it.
And I imagine, especially when you’re deploying to financial services there can sometimes be challenges integrating into their systems. Can you talk about some of the headwinds that are sometimes experienced at that customer level?
Integration is always a topic and the bigger the organization, the bigger the integration needs.
And of course, machine learning lives off data at data and organizations is stored in various silos. They can be very simple silos like some of these computers or they can be very sophisticated locations like a data lake or a data warehouse.
Getting the data from A to B that’s the job of integration. And so the B, in this case, might be our platform to help train the machine learning models. So yeah, integration into the data landscape in an organization is essential for machine learning to succeed.
That’s fascinating. Kevin Schawinski is the co-founder and CEO of Modulos AG.
Kevin, thanks for joining us.
Thanks for having me.
We are very pleased to be joined by Andreas Rath, the founder, and CEO of ONDEWO , a conversational AI company based out of Vienna, Austria.
Andreas, welcome to the podcast.
Thanks a lot for having me; very much looking forward to the discussion.
So how did you become an entrepreneur and why did you choose AI as a subject to tackle?
So I am technical mathematics. PhD with a focus on artificial intelligence. I was in research for six years…published 30 papers or so in the area of human machine interaction. It always fascinated me even 15 years ago, how artificial intelligence can support people in doing their daily jobs.
After my research I joined a large international management consultancy company, McKinsey. I digitized over 18 companies in 13 different countries on three continents. And I just love how much software and AI can help us to automate processes. And this was also the reason why I founded my company in 2017 with the passion to teach machines, to be more intelligent so they can help us better.
Every business begins with a problem that you’re trying to solve. What problem did you identify that needed to be solved?
The problem we identified is actually very simple. When you call a company, usually what happens is you’re stuck in the waiting line, on the phone, or you cannot reach them or an agent can not tell you on the phone, how you get things done, how you can register something or cancel something.
And we felt the need – there is something we could do to make a service available 24×7 for the customer. So this was one reason. The second reason was when we dig deeper in this problem, we found out it’s actually an expectation management issue.
The customer has the expectation to be served 24×7 with a very high quality of service. However, call centers or customer support centers are pressured by cost reduction. So lower cost. And also, the skills are not there, which means they have a 30 to 40% churn rate on the employees. So just imagine after every year or so, 30 to 40% or even more in some areas of the world are leaving your company.
So the equation didn’t make sense. Higher customer expectation on call quality of service availability, at low cost with low skills. That doesn’t work out. So this was the problem we are trying to solve with so-called AI agents that pick up the phone or text you fully, automatically.
Tell me more about the customers you serve and the kinds of solutions that you’re delivering to them.
Our main customers, are IT service providers who operate call centers for their customers.
So we are a B2B product company. So we provide an all-in-one, end-to-end solution to automate inbound and outbound phone calls and check messages in 10 languages at the moment, focusing on the European market with 30 beautiful sounding voices on the phone and via chat or voice assistant.
And with these solutions, we take to the service providers and teach them how to use it. And we leveraged their expertise and process optimization in automating call centers, making more sufficient to actually scale our solution, which means when they know how to operate our low code or no code solution, it makes it very easy and very fast to optimize their costs and increase their service quality, the customer experience on the phone, and also the service availability.
So we’re really kind of targeting large call centers that have thousands of calls everyday, to be automated.
You told me something in our prep discussion that I thought was very unique. You talked about beautiful voices. Tell me again what voices you have incorporated into your solution and why?
We found out that the adoption rate of these AI systems have one key factor, which is how well the voice of the AI on the phone sounds to people. And as you know, when you meet friends or new people, you always listen to the voice and you judge very fas how do like this person or not. So in order to improve this experience and this adoption rate of AI systems, we started to produce voices entire legs. So for example, we built the first Bavarian AI voice in the world three weeks ago. And probably you remember October Fest and how they speak there.
And this voice is actually talking Bairisch, so Bavarian. And therefore we think that the adoption is much higher. We also produce voices that sound very natural and human-like. So we are not using professional speakers for our training data. We are using normal people who have a beautiful sounding voice. Maybe they have a little bit of dialect or a tendency. For example, we have one German speaker, she’s a female, and she has a British dialect a little bit of German and you feed it a little bit. So it becomes very authentic or an Austrian male who has a little bit the Styrian influence.
So, you actually feel that this person is not speaking professional, German word by word, but you feel a little bit influenced and therefore you have, you find it very sympatico on the phone and therefore the, you feel, okay, this might be a really human being and I can trust, and I can feel comfortable talking to the AI on the phone.
That makes us very special because most of the players out there – especially large cloud providers – have also beautiful sounding voices, but more like these professional types like Google assistant…Siri…they sound very beautiful, of course, however, kind of too perfect. I would say, and we as humans, we are not perfect and this is what we actually love when we interact with people. And this is what we try to replicate here and use.
That’s a fascinating approach that you’ve taken now every emerging business wants to break into large customers. Getting those big enterprise customers in your portfolio is incredibly important for the health and growth of your business.
Tell us about some of the challenges that ONDEWO has faced in reaching that scale, and how you have addressed some of those challenges.
Yeah, that’s a very good question and it was very difficult, I have to admit the cost is large. Corporations have a very high bar in terms of standards you need to fulfill in terms of quality of your AI solution, your models, for example, speech recognition quality, automation, the capability of complex dialogues, and conversational processes. And also how beautiful and natural the voices.
As an example, we did a case with one of our it service providers, which is Atos, the large IT corporation. We’re building a use case for inbound and outbound call automation for banks. And the it support area.
The Atos project actually had challenges. The first challenge was to make AI work in terms of speech recognition and dialogue. The second was the integration capabilities and the third one was to actually testing and real infrastructure environment. The first one we saw worked very nicely in collaboration with our AI team.
The second one, we had no solutions for – it’s the integration part because ONDEWO is a product company focusing on AI technologies, but not the integration technologies. So, we were looking for a partner who can handle real time conversation integration. For backend systems like ServiceNow, or Jira and so on and now we found Qorus, which is one of our partners to help us very easily, very fast, consistently data stable, integrate our solution into a banking IT service support application in real time. And real time is the key because the conversation on the phone needs to happen in real time.
So we had to look for a solution which also supports this realtime element and which Qorus did very nicely.
It’s fascinating. And it’s been really such a pleasure to learn about you, ONDEWO, and of course, dialects, which I think is so exciting.
And I look forward to having that first experience when I’m actually hearing someone on the phone, I should say some computer, some machine talking to me in a dialect that would make my face brighten up and smile.
Andreas, thank you so much for your time today. It’s been such a pleasure to have a great AI Summit and London Tech Week and all the best to you and everyone at ONDEWO.
Thanks a lot, Greg. All the best.
We are very pleased to welcome Kuba Krchak from Mamma AI, who’s joining us on the podcast. Kuba, welcome.
Thanks for having me, Greg.
I know from our earlier conversations, you have a most fascinating journey. Why don’t you take us through a bit of your academic and professional history leading up to this point?
So I have been actually already studying artificial intelligence at the turn of the millennium. And I would say it was before the neural networks were cool or maybe they were cool, but they were not so useful then.
So after my master’s in computer science, I joined IBM and I worked on artificial intelligence, speech recognition and on various Watson APIs. That is where I met many of my other colleagues that together we founded Mamma AI.
Where’d you come up with that name?
So of course mama is mother, right? So it’s this foundational cross culture, cross language sound that they share together. So of course our human computer interaction background brought us to something that’s very foundational to all human speech.
And then that sounds “mama” is for many humans on the planet – I would say maybe billions of them – the first sound that they ever say in their life. So that’s one of the motivations and other motivation is that AI computers is kind of cold, it’s very technical and we wanted to have some warm archetype to pair with this cold and technical AI business.
So that’s why we thought let’s put the mama there. It will better represent the, the warmth and the love and these kinds of things. And lastly, as I said, we work a lot in the communication of computer and humans. So the mama also goes for men and machine. So a lot of thinking into it, many different aspects of why we liked the name.
When you began the mama AI, what was the core problem you are trying to solve?
We knew that AI is very powerful. And when we look around, there’s a large amount of companies that are not leveraging AI in any way. The real AI use for business is just scratching the surface of possibilities. So we wanted to be there for our customers, for the companies out there, to help them really use AI for their advantage…generate additional revenue, save money, and open up people so they can do more innovative work because the more mundane tasks are done by AI and so on. So this kind of basic help for anyone who wants to get AI, really working for them.
What types of companies are your customers?
One of the biggest sectors that use this AI right now where there’s biggest proliferation of AI use is the financial business and insurance and business. So for us, it is similar. These are the most advanced AI users and we have the largest footprint in this vertical.
But there is a set of other verticals where there’s also AI finding its way. So it could be retail, e-commerce business, utilities business…communications business. One area that we also have found quite a lot of interest in AI and are using it very well is biomedicine, biochemistry. These are some of the verticals where we have been able to successfully help with AI.
So that’s a very broad reach, which is exciting. And obviously, there are some small and mid-size companies across all of those categories, but equally, very large customers. You talk about utilities, you’re talking about biomedicine, pharmaceutical, perhaps you’re talking about big, big enterprises.
When you go to deploy your solutions at some of these larger customer sites. What do you find are some of the key challenges that a successful deployment has?
That’s an excellent question and I would say there is one quite fundamental enabler for AI. For AI to work, it needs to have data and the data needs to be well organized. And it needs to be accessible, right? It’s this part of the well-organized.
So this is something that across all of our clients is what we are often struggling with. That there hasn’t been yet a full digital transformation process done and our clients are possibly not saving their data or they are not having the data available easily through some rest APIs that we could use so this often is like a total blocker for us.
We sometimes say without IA there’s no AI, right. There needs to be the intelligent architecture for the AI to be able to thrive.
That is absolutely fascinating.
Kuba Krchak fromMama AI, it’s been such a pleasure talking to you, learning from you. Wishing you much more success in the rest of this year and years to come. Thank you so much for joining us.
Thanks for having me, Greg and some of our team will be at the AI Summit in London so if you want to know more about my AI team, feel free to find us there.
Yes, that’s fantastic. We are looking forward to AI Summit and people should absolutely come and find Mamma AI.
Thank you, Kuba.
Thanks a lot, Greg.
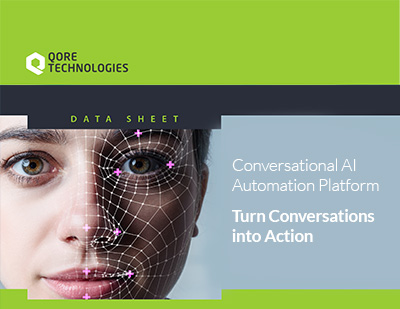